New global study surveys the state of business AI
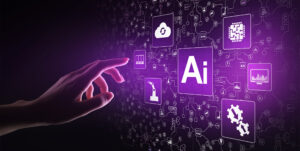
ClearML has announced new research findings from a global AI survey recently conducted with FuriosaAI and the AI Infrastructure Alliance (AIIA). The new report, called “The State of AI Infrastructure at Scale 2024: Unveiling Future Landscapes, Key Insights and Business Benchmarks” includes responses from AI/ML (artificial intelligence/machine learning, a branch of AI) and other technology leaders at 1,000 companies of various sizes across North America, Europe and Asia Pacific. The report focuses on how executives are building their AI infrastructure; the critical benchmarks and key challenges they face; and how they rank priorities when evaluating AI infrastructure solutions against their business use cases. The report dives into respondents’ current scheduling, computations and AI/ML needs for training and deploying models and AI framework plans for 2024-25.
One of the primary drivers propelling hypergrowth in the AI infrastructure market is the realization among organizations of how AI can drive their operational efficiency and workforce productivity as the leading business use case. Companies are recognizing the need for Gen AI (generative artificial intelligence) solutions to extract actionable insights from their internal knowledge bases and plan to deploy Gen AI to boost their competitive edge, enhance knowledge worker productivity and affect their bottom line.
As companies navigate the AI infrastructure market, they are seeking clarity, peer insights and reviews, as well as industry benchmarks on AI/ML platforms and compute. To understand executives’ biggest pain points in moving AI/ML to production, this survey examined not only model training, but also model serving and inference.
“Our research shows that while most organizations are planning to expand their AI infrastructure, they can’t afford to move too fast in deploying Generative AI at scale at the cost of not prioritizing the right use cases,” said Noam Harel, ClearML’s chief marketing officer and general manager, North America in Montreal. “We also explore the myriad challenges organizations face in their current AI workloads and how their ambitious plans for the future signal a need for highly performant, cost-effective ways to optimize GPU (Graphics Processing Unit) utilization (or find alternatives to GPUs), and harness seamless, end-to-end AI/ML platforms to drive effective, self-serve compute orchestration and scheduling with maximum utilization.”
“There are lots of claims about how businesses are addressing their rapidly evolving AI infrastructure resource needs and incorporating Generative AI into their products. This report provides hard data to answer these questions,” said June Paik, FuriosaAI’s CEO. “In particular, it shows how businesses are actively looking for new, cost-effective options for inference compute. We’re excited to see that our second-gen product, launching later this year, directly addresses one of the top concerns cited in the report.”
Key insights included:
- 96% of respondents plan to expand their AI computation infrastructure with availability, cost and infrastructure challenges weighing on their minds, with 40% considering more on-premise and 60% considering more cloud, as they are looking for flexibility and speed. The top concern for cloud computation is wastage/idle costs.
- 95% of executives reported that having and using Open Source technology is important for their organization. Of the respondents, 96% are focused on customizing Open Source models. PyTorch is their framework of choice.
- A staggering 74% of companies are dissatisfied with their current job scheduling and orchestration tools and face computation resource on-demand allocation and team productivity constraints. Of those surveyed, 74% see value in having computation and scheduling functionality as part of a single, unified AI/ML platform (instead of cobbling together an AI infrastructure tech stack of stand-alone point solutions). But only 19% of respondents actually have a scheduling tool that supports the ability to view and manage jobs within queues and effectively optimize GPU utilization. It is important to note that 93% of surveyed executives believe that AI team productivity would substantially increase if computation resources could be self-served.
- Optimizing GPU utilization and GPU partitioning are major concerns, with the majority of GPUs underutilized during peak times. Of respondents, 40%, regardless of company size, are planning to use orchestration and scheduling technology to maximize the current computation investments of their existing AI infrastructure. Only 42% of companies have the ability to manage Dynamic MiG/GPU partitioning capabilities to optimize GPU utilization.
- Cost is the key buying factor for inference computation. To address GPU scarcity, 52% of respondents reported actively looking for cost-effective alternatives to GPUs for inference in 2024 as compared to 27% for training. Of those surveyed, 20% were interested in cost-effective alternatives to GPU but were not aware of existing alternatives. This indicates that cost is a key buying factor for inference solutions. We expect that while industries are still in early days for inference, the demand for cost-efficient inference computation will grow.
- The biggest challenges for computation were latency, followed by access to computation and power consumption. Over half of respondents plan to use language models (like LLama), followed by embedding models (BERT and family), 26% in their commercial deployments. Mitigating computation challenges will be essential in their plans.
Download a free copy of the global survey report here.